Five generative AI use cases for the financial services industry Google Cloud Blog
You can foun additiona information about ai customer service and artificial intelligence and NLP. Transformers form the basis of the large language models we know today and represent a significant improvement over previous architectures in their ability to understand and generate human language, which word-based models could not do. Meanwhile, finance research has progressed in the subfield of natural language processing, an area in which ML techniques are turned on language itself to mine information from text. Early adopters of language tools included Shanghai Jiao Tong University’s Feng Li (a graduate of Booth’s PhD program), who in 2008 studied the relationship between the readability of 10-K filings and corporate performance.
Fraud management powered by AI raises security standards, safeguards client assets, strengthens brand image, and reduces the operational strain on the investigation teams. For example, BloombergGPT can accurately respond to some finance related questions compared to other generative models. This automation not only streamlines the reporting process and reduces manual effort, but it also ensures consistency, accuracy, and timely delivery of reports. Banks want to save themselves from relying on archaic software and have ongoing efforts to modernize their software. Enterprise GenAI models can convert code from old software languages to modern ones and developers can validate the new software saving significant time. While there are many different approaches to AI, there are three AI capabilities finance teams should ensure their CPM solution includes.
This is the technology that underpins image and speech recognition used by companies like Meta Platforms (META 0.19%) to screen out banned images like nudity or Apple's (AAPL -0.86%) Siri to understand spoken language. MSCI is also partnering with Google Cloud to accelerate gen AI-powered solutions for the investment management industry with a focus on climate analytics. To fully understand global markets and risk, investment firms must analyze diverse company filings, transcripts, reports, and complex data in multiple formats, and quickly and effectively query the data to fill their knowledge bases.
For Generative AI, this translates to tools that create original content modalities (e.g., text, images, audio, code, voice, video) that would have previously taken human skill and expertise to create. Popular applications like OpenAI’s ChatGPT, Google Bard, and Microsoft’s Bing AI are prime examples of this foundational model, and these AI tools are at the center of the new phase of AI. That is where AI in finance has really made a difference, aiding in trading. AI algorithms are used to automate trading strategies by analyzing market data and executing trades at optimal times. AI systems browse through reams of market data at an incredible speed and with high accuracy, sensing trends and making trades almost as fast as they can be. It's like an Avengers-level calculator that gets to predict the movement of the markets very accurately.
This enables more personalized interactions, faster and more accurate customer support, credit scoring refinements and innovative products and services. AI-driven data science can enhance decision-making in real-time, while automation provides cost savings and faster transactions. By deploying accurate algorithms and predictive models with new technologies in software, financial institutions and businesses can automate their operations and gain valuable insights into customer behavior. DataRobot provides machine learning software for data scientists, business analysts, software engineers, executives and IT professionals.
Europe and emerging markets in Asia and South America will follow, with moderate profits owing to fewer and later investments (PwC 2017). Automating middle-office tasks with AI has the potential to save North American banks $70 billion by 2025. Further, the aggregate potential cost savings for banks from AI applications is estimated at $447 billion by 2023, with the front and middle office accounting for $416 billion of that total. Read on to learn about 15 common examples of artificial intelligence in finance, how financial firms are using AI, information about ethics and what the future looks like for this rapidly evolving industry. Has your bank ever called you to verify account activity on your credit card?
What impact does AI have on financial services customer support?
What was the highest-performing marketing campaign in Q4 — and how can we make it even more impactful? AI can analyze demand, marketing, and sales data in context to determine the most successful marketing campaign and provide recommendations to maximize the impact of that campaign. While it is crucial to talk about the major benefits of AI in finance, we must not overlook the possible challenges and risks it can pose. Now, with the availability of Artificial Intelligence-driven tools, there are customized retirement calculators and planning strategies through which individuals can easily plan their future.
AI technologies advanced significantly to detect fraudulent actions and maintain system security. Using AI for fraud detection can also improve general regulatory compliance matters, lower workload, and operational costs by limiting exposure to fraudulent documents. In a case study2, DZ Bank has reduced the workload of security operations teams by 36x. AI can analyze relevant financial information and provide insights about financials by leveraging techniques like machine learning and natural language processing. Instead of conducting numerous calculations in spreadsheets or financial documents, AI can rapidly handle large volumes of documents and deliver insights without missing an important point. Advanced machine learning algorithms analyze vast datasets to identify unusual patterns and behaviors indicative of fraudulent activities.
What Is Artificial Intelligence (AI)? - Investopedia
What Is Artificial Intelligence (AI)?.
Posted: Tue, 09 Apr 2024 07:00:00 GMT [source]
By spotting unusual patterns and identifying correlating trends, AI can identify both risks and opportunities in performance data. Human-identified data trends tend to be linear and one-dimensional in scope. AI can identify correlations between diverse data types at a much more sophisticated level of analysis. For example, the AI could tell you the trajectory of sales and identify the factors driving sales in that direction and show you how to change drivers to influence the trajectory of sales. The capability of AI to assess and anticipate patterns plays a vital role in managing risks.
Gen AI leverage in finance allows finance businesses to gain the upper hand in making insightful decisions based on real-time behavioural analysis. The novel content generation fits the finance industry well, enabling impressive portfolio creation, optimizing strategy, and improved fraud detection. AI in finance automates transactions, enhances data analysis, improves customer service, and boosts security through fraud detection and risk management systems. In fact, a recent study found that AI algorithms outperformed traditional rule-based systems by up to 20% in detecting fraudulent credit card transactions. Additionally, AI-based fraud detection can process vast amounts of data in real-time, enabling financial institutions to detect suspicious activities with speed and accuracy.
The tool taps into a vast library of documents to provide users with instant, accurate insights. Such tools use a person's current data to prepare a plan under his/her name—much easier and effective in terms of retirement planning management. AI can help optimize contributions to a Roth account, considering factors like current income, tax implications, and long-term financial goals. These tools provide a comprehensive approach to retirement planning, incorporating various account types and investment strategies. There are a lot of applications for AI in banking and finance that are already being used to enhance daily processes and provide a better experience to users. These technologies are not only transforming how financial institutions operate but are also setting new standards for efficiency and customer engagement.
AI really burst into public consciousness in 2022, when OpenAI introduced ChatGPT, the generative AI tool that can conduct conversations, write computer code, compose music, craft essays and supply endless streams of information. The arrival of generative AI has raised worries that chatbots will replace freelance writers, editors, coders, telemarketers, customer-service reps, paralegals and many more. AI researchers are still debating how best to evaluate the capabilities of the latest generative AI technology and how it compares to human intelligence. There are tests that judge AI on solving puzzles, logical reasoning or how swiftly and accurately it predicts what text will answer a person's chatbot query. Those measurements help assess an AI tool's usefulness for a given task, but there's no easy way of knowing which one is so widely capable that it poses a danger to humanity.
Additionally, unforeseen developments in AI technology also mean that firms must continuously adapt, making long-term planning difficult. The high investment required for AI technology means that small businesses may find it difficult to allocate funds. Artificial intelligence in accounting and finance firms will boost efficiency and enable firms to offer more value-added services.
Operations
A good example is when its AI claims processing agent (AI-Jim) paid a theft claim in just three seconds in 2016. The company reiterates that currently, it can settle around half of its claims by employing AI technology. Yet another good example is the Bank of England (BoE) employing AI in credit risk management in the areas of pricing and underwriting of insurance policies. The business leaders within the institution reiterate the edge of AI algorithms over traditional models, offering an unmatched level of sophistication. Jumio’s KYX platform helps businesses establish trust with online customers.
Demo your latest financial technology to 2,000+ senior execs from financial institutions, VC’s and more. Chicago Booth Review
Research driven https://chat.openai.com/ insights on business, policy, and markets. We accept payments via credit card, wire transfer, Western Union, and (when available) bank loan.
Likewise, the feed-forward neural network effectively approximates the daily logarithmic returns of BTCUSD and the shape of their distribution (Pichl and Kaizoji 2017). In this paragraph, we shortly illustrate some relevant characteristics of our sub-sample made up of 110 studies, including country and industry coverage, method and underpinning theoretical background. Table 2 comprises the list of countries under scrutiny, and, for each of them, a list of papers that perform their analysis on that country. We can see that our sample exhibits significant geographical heterogeneity, as it covers 74 countries across all continents; however, the most investigated areas are three, that is Europe, the US and China.
This means we can respond more quickly to market changes or operational demands. This agility is crucial in the fast-paced world of finance, where conditions can change rapidly. Taking a glance at the plethora of financial regulations could sometimes be overwhelming.
Choose the Right Generative AI Models
These predictions help financial experts to identify risks and ensure better information for future planning. As seen before, AI can perform advanced fraud detection and doing so it can drastically challenge ai in finance examples financial crime and spot anomalous activity. For instance, banks can use AI forecasting models to estimate future loan default rates to better measure risk exposure and provision capital reserves adequately.
- By streamlining and consolidating tasks and analyzing data and information far faster than humans, AI has had a profound impact, and experts predict that it will save the banking industry about $1 trillion by 2030.
- While there are many different approaches to AI, there are three AI capabilities finance teams should ensure their CPM solution includes.
- To build Treemaps, we utilized squarified treemapping algorithm, which is widely accepted by a broad audience, especially in financial contexts.
These tools include everything from intelligent automation to machine learning, natural language processing, and Generative AI, and they present new opportunities, possible benefits, and many emerging risks for finance and accounting. AI is revolutionizing how financial institutions operate and fueling startups. AI models execute trades with unprecedented speed and precision, taking advantage of real-time market data to unlock deeper insights and dictate where investments are made. AI is also changing the way financial organizations engage with customers, predicting their behavior and understanding their purchase preferences.
We are granted with research funds by our institution which would allow us to cover the publication costs. 2 provides a visual representation of the citation-based relationships amongst papers starting from the most-cited papers, which we obtained using the Java application CiteSpace. After that, focussing on the more pertinent (110) articles, we checked the journals in which these studies were published.
- Yes, this is annoying for some, but the process will become more accessible and more pleasant over time.
- AI is changing the game for financial customer service, making it faster, smoother, and much more convenient.
- When we talk to digital assistants, use autocomplete, incorporate process automation tools, or use predictive analytics, we are using AI.
Using AI to unlock the potential in the finance sector offers limitless possibilities. It's a journey that financial chiefs need to consider and open the door to more innovations. Here are a few examples of companies using AI and blockchain to raise capital, manage crypto and more.
For each journal, we also report the total number of studies published in that journal. A proactive approach significantly strengthens the security and reliability of financial operations. It ensures the protection of sensitive information and transactions from cyber threats.
A valuable research area that should be further explored concerns the incorporation of text-based input data, such as tweets, blogs, and comments, for option price prediction (Jang and Lee 2019). Since derivative pricing is an utterly complicated task, Chen and Wan (2021) suggest studying advanced AI designs that minimise computational costs. Funahashi (2020) recognises a typical human learning process (i.e. recognition by differences) and applies it to the model, significantly simplifying the pricing problem. In the light of these considerations, prospective research may also investigate other human learning and reasoning paths that can improve AI reasoning skills.
This approach is similar to some of the “individualized data” use cases of AI in insurance. For instance, Progressive, a leading insurance company in the USA, collects data about individual drivers to predict their risk of accidents better. We also built robust compliance frameworks, including the Financial Conduct Authority (FCA), to handle the complex regulatory landscape, ensuring timely updates and adjustments in response to new FCA directives. Complying with legal and regulatory requirements is essential for the responsible and compliant use of AI in spend management.
He found that companies with longer and more difficult-to-read reports tended to have poorer earnings. As you reflect on these examples, consider how AI could address your business’s unique challenges. Whether optimizing operations, enhancing customer satisfaction, or driving cost savings, AI can provide a competitive advantage. In the dental care field, VideaHealth uses an advanced AI platform to enhance the accuracy and efficiency of diagnoses based on X-rays. It’s particularly powerful because it can detect potential issues such as cavities, gum disease, and other oral health concerns often overlooked by the human eye. Another challenge is that AI needs help with contextual understanding to reduce errors in complex accounting tasks.
Artificial Intelligence automatically undertakes many financial activities and optimizes them; hence, this brings down operational costs. This fall in expenses directly translates into savings for the businesses and, therefore, more affordably priced services to customers. For the successful development and deployment of Gen AI applications, artificial intelligence consulting companies will help you identify which Gen AI use case is great for achieving AI objectives. It’s followed by selecting and customizing Gen AI models for your finance project. Creating financial reports is quite a task as it involves collecting data from diverse sources and presenting them in a particular format. Gen AI makes it effortless by analyzing data collected from financial institutions, investors, and regulatory bodies.
Artificial Intelligence in finance greatly enhances operational efficiency through the automation of routine tasks and the quick processing of information. Increased speeds, such as in decision-making and task management, will help reduce wait times and increase overall productivity. These systems are more than capable of analyzing and detecting unusual patterns that may indicate fraudulent activity. Machine learning models can learn from historical fraud data to predict and prevent future occurrences. Examining trends and flagging suspicious behavior, AI performs the role of an alert guard in securing financial transactions.
While gen AI is still in its early stages of deployment, it has the potential to revolutionize the way financial services institutions operate. The last group studies intelligent credit scoring models, with machine learning systems, Adaboost and random forest delivering the best forecasts for credit rating changes. These models are robust to outliers, missing values and overfitting, and require minimal data intervention (Jones et al. 2015). As an illustration, combining data mining and machine learning, Xu et al. (2019) build a highly sophisticated model that selects the most important predictors and eliminates noisy variables, before performing the task. The second sub-stream focuses on mortgage and loan default prediction (Feldman and Gross 2005; Episcopos, Pericli, and Hu, 1998). All the forecasting techniques adopted (i.e. supervised machine learning and ANNs) outperform linear models in terms of efficiency and precision.
We leveraged React for highly responsive web interfaces and employed React Native with Expo for a seamless cross-platform experience. Simform developed an online P2P (peer-to-peer) lending platform
It directly connects borrowers with individual investors, sidestepping traditional intermediaries like banks. By completing KYC in minutes rather than days, AI technologies significantly reduce costs, minimize errors, and improve customer satisfaction.
To do this, the artificial intelligence model analyzes text to identify patterns and keywords. By working with supplier-specific models, Yokoy’s AI-engine is able to process invoices with much higher accuracy rates than other invoice automation apps on the market. Now let’s take a closer look to some specific AI-powered automation scenarios that apply to the spend management process. We’ll start with the spend management process, as this is our main area of expertise.
The multinational financial services company is committed to serving customers best and revolutionizing services with Gen AI’s transformative force. They have implemented predictive banking functionality to provide personalized financial guidance to customers depending on tailored account insights. As adoption increases, the future of AI in finance includes fraud detection, customer service automation, and improved credit scoring for making better credit decisions. The future of AI in financial services looks promising with the potential to further revolutionize the industry. As technology advances, AI is expected to become more sophisticated, with deeper integration into all aspects of financial operations from personalized banking to more secure and efficient regulatory compliance.
AI-driven risk management tools assess creditworthiness more accurately than traditional methods. This real-time analysis helps adjust trading strategies and optimize risk management practices. It also ensures proactive responses to evolving market dynamics and enhances decision-making processes. Further, the use of NLP can aid text mining and analysis of social media data such as tweets, Instagram posts, and Facebook posts, which impact trading decisions. Yet another exciting facet is the use of reinforcement learning-based AI models, which can adjust to dynamically changing market conditions. Thus, AI/ML models enable traders to make more informed decisions, manage risk, and maximize profits.
American Express’ AI decision engine analyzes over $1 trillion in transactions annually, minimizing fraud. AI significantly reduces operational overheads by automating labor-intensive and repetitive tasks like data entry, document processing, and reconciliation, ultimately leading to cost savings. Generative AI is expected to add new value of $200-$340 billion annually (equivalent to 9 to 15 percent of operating profits) for the banking sector. Automating processes is probably the most common use case of artificial intelligence in the finance industry, as this technology has evolved enough to be able to take over most of the tasks traditionally performed by humans.
Legal transparency in AI finance: facing the accountability dilemma in digital decision-making - Reuters
Legal transparency in AI finance: facing the accountability dilemma in digital decision-making.
Posted: Fri, 01 Mar 2024 08:00:00 GMT [source]
Artificial intelligence can be used to analyze large datasets and identify fraudulent activities – such as credit card fraud or money laundering – in real-time. Overall, AI can help with process automation, streamlining the VAT reclaim process, reducing the time and resources required to manage tax reclaims, and minimizing the risk of human errors. This can lead to significant cost savings for companies and provide greater accuracy and efficiency in the VAT reclaim process. The cost-saving potential of artificial intelligence only adds to its appeal to banks and other financial companies. If you're looking for an investment opportunity, consider some of the stocks above, as well as other AI stocks or AI ETFs if you're looking for a broad-based approach to the sector.
This predictive capability allows UPS to proactively mitigate risks by rerouting at-risk packages to secure locations, such as The UPS Store or UPS Access Points, reducing the likelihood of theft. Let’s explore three real-world examples of companies powerfully leveraging AI. Integrating AI into accounting brings unique challenges that can be daunting, even for the most tech-savvy firms. Despite its numerous benefits, incorporating artificial intelligence in accounting is challenging.
Financial services leaders are no longer just experimenting with gen AI, they are already way building and rolling out their most innovative ideas. You can start implementing these use cases using Google Cloud’s Vertex AI Search and Conversation as their core component. With Vertex AI Search and Conversation, even early career developers can rapidly build and deploy chatbots and search applications in minutes. Open access funding provided by Università Politecnica delle Marche within the CRUI-CARE Agreement.
Fraud detection and risk management
AI dramatically accelerates customer service and response times in finance by processing information at speeds far beyond traditional methods. This rapid processing capability allows financial institutions to offer instant financial services such as real-time transaction processing, immediate customer feedback, and quick resolution of inquiries and issues. Traditionally, financial companies based their decisions on past data and gut feelings.
These adaptive AI systems enhance their predictive capabilities by continuously learning from new data. This provides financial institutions with robust tools to mitigate risks and safeguard Chat GPT assets. Financial institutions are deploying real-time monitoring systems powered by AI. These systems detect and flag suspicious transactions, significantly reducing fraud.
Learn how to transform your essential finance processes with trusted data, AI insights and automation. Explore what generative artificial intelligence means for the future of AI, finance and accounting (F&A). The use of AI in finance requires monitoring to ensure proper use and minimal risk. Proactive governance can drive responsible, ethical and transparent AI usage, which is critical as financial institutions handle vast amounts of sensitive data. The financial industry encompasses several subsectors, from banking to insurance to fintech.
There are too many decisions that require personal judgment for humans to be fully replaced by AI in investing. However, the cost-saving potential of artificial intelligence allows for decisions to be made more rapidly and inexpensively, and it could eliminate lower-level work in areas like research and underwriting. Given the wide range of applications, it is likely that AI will continue to grow throughout the finance industry in the future. AI is being used in finance in a variety of ways, including investing, lending, fraud detection, risk analysis for insurance, and even customer service. Artificial Intelligence (AI) in finance refers to the use of machine learning to enhance how financial institutions analyze and manage investments.
Let’s explore a few use cases and success stories before delving into actionable mitigation strategies inspired by these illustrations. As highly regulated industry players, banks get regular requests from regulators. Explore how generative AI legal applications can help take actions against fraudulent activities. The AI would instantly pull results from your performance data and organize it into a report that is ready for analysis. It’s the beginning of Q2, and you need to create a plan for a product line in the EMEA. By analyzing the region’s data, the product line sales history, and market information, AI can determine the business drivers influencing sales so you can apply that insight to your sales plan and strategy for the coming quarter.
It's a highly competitive industry, as banks and other operators constantly seek an edge over one another. In July 2024, Robinhood acquired Pluto Capital, which is a free trading platform that's supported by LLM and other AI-powered tools to help users create and automate trading strategies, for an undisclosed sum. In addition to chatbots, banks use AI to help recommend products for customers and manage money.
The technologies are helping the financial sector to achieve its goals of personalized and reliable services meeting the needs and expectations of its customers. Thus, customers get faster and more accurate responses to their queries and requests through channels such as voice assistants, chatbots, and email. Consequently, customer sentiment and feedback are enhanced, increasing customer trust and satisfaction. In the past five years, researchers have embraced ML to solve finance problems. In 2020, Booth PhD student Shihao Gu, Yale’s Bryan T. Kelly, and Booth’s Dacheng Xiu summarized the performance of diverse ML models when applied to finance.
With the scope of preventing further global financial crises, the banking industry relies on financial decision support systems (FDSSs), which are strongly improved by AI-based models (Abedin et al. 2019). One of the most significant business cases for AI in finance is its ability to prevent fraud and cyberattacks. Consumers look for banks and other financial services that provide secure accounts, especially with online payment fraud losses expected to jump to $48 billion per year by 2023, according to Insider Intelligence.
AI should be a powerful tool that makes undiscernible information discernible. EY writes that ultimately, finance teams need to see AI as a collaboration where AI can do the repetitive work and finance teams can do the strategic work. “While AI can process vast amounts of data at a rapid pace, it lacks the critical thinking and decision-making capabilities of people. AI reduces errors to a large extent and increases accuracy by deriving data-driven insights and predictive models. This leads to making sure that one has more secure financial decisions and operations, hence reducing possibilities of errors through human failure.
記事を書いたライター
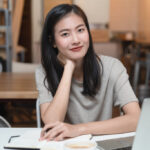
- BANANA GA.MEライター
- 食品と栄養学に関連する記事を執筆する経験豊富なライターです。彼女は栄養学の学位を持ち、バナナを含む果物の健康効果や栄養価について幅広い知識を持っています。また、彼女は食品業界での経験もあり、持続可能な食品生産や食品安全性に関する洞察を提供できます。
ライターの新着記事
FOOD2024.12.27BANANA 551: ハーゲンダッツ豆乳バナナショコラ
FOOD2024.12.17BANANA 550: バナナの王様 甘熟王バナナクリーム&ホイップ
FOOD2024.12.07BANANA 549: ロングスティック バナナクリーム
FOOD2024.11.27BANANA 548: SPARKLING BANANA